Intel published an interesting lbog on "How to Get Started as a Developer in AI". As AI will become a central part of our life, developers who will jump in the AI train early will have a clear edge.
The article starts with a definition of AI
Sense—Identify and recognize meaningful objects or concepts in the midst of vast data. Is that a stoplight? Is it a tumor or normal tissue?
Reason—Understand the larger context, and make a plan to achieve a goal. If the goal is to avoid a collision, the car must calculate the likelihood of a crash based on vehicle behaviors, proximity, speed, and road conditions.
Act—Either recommend or directly initiate the best course of action. Based on vehicle and traffic analysis, it may brake, accelerate, or prepare safety mechanisms.
Adapt—Finally, we must be able to adapt algorithms at each phase based on experience, retraining them to be ever more intelligent. Autonomous vehicle algorithms should be re-trained to recognize more blind spots, factor new variables into the context, and adjust actions based on previous incidents.
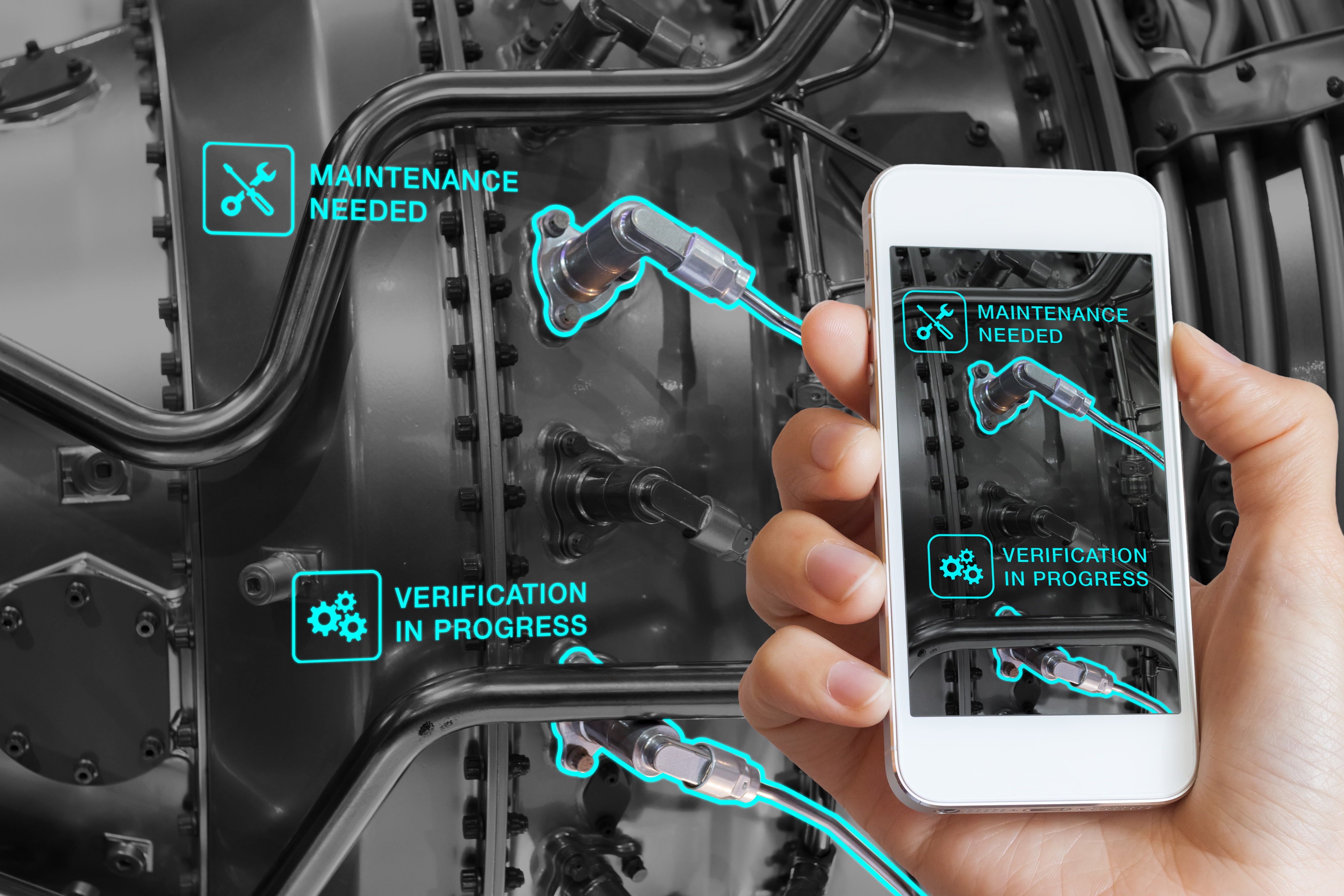
The article continues with a typical machine learning workflow:
Data Acquisition—First, you need huge amounts of data. This data can be collected from any number of sources, including sensors in wearables and other objects, the cloud, and the Web.
Data Aggregation and Curation—Once the data is collected, data scientists will aggregate and label it (in the case of supervised machine learning).
Model Development—Next, the data is used to develop a model, which then gets trained for accuracy and optimized for performance.
Model Deployment and Scoring—The model is deployed in an application, where it is used to make predictions based on new data.
Update with New Data—As more data comes in, the model becomes even more refined and more accurate. For instance, as an autonomous car drives, the application pulls in real-time information through sensors, GPS, 360-degree video capture, and more, which it can then use to optimize future predictions.
The first potential application of Articifial Intelligence in the manufacturing industry is related to the design algorithms to anticipate repairs and improve preventive maintenance. We can also think of performance improvement machine learning over time to determine which products to transform on which line and the ideal production sequence to optimize ressources.
SOURCE: https://software.intel.com/en-us/articles/how-to-get-started-as-a-developer-in-ai?